Best Practice: Assure
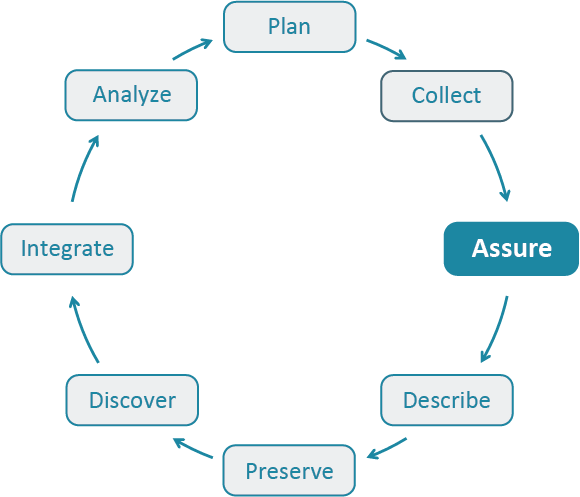
Select a Best Practice below to learn more about the “Assure” stage in the Data Life Cycle.
What is the “Assure” stage?
Employ quality assurance and quality control procedures that enhance the quality of data (e.g., training participants, routine instrument calibration) and identify potential errors and techniques to address them.
More information can be found in the Best Practices Primer.
-
Communicate data quality
Information about quality control and quality assurance are important components of the metadata: (click for more)
Confirm a match between data and their description in metadataTo assure that metadata correctly describes what is actually in a data file, visual inspection or analysis should be done by someone not otherwise familiar with the data and its format. This will assure that the metadata is sufficient to describe the da... (click for more)
Tags: assure data consistency describe documentation metadata quality
Consider the compatibility of the data you are integratingThe integration of multiple data sets from different sources requires that they be compatible. Methods used to create the data should be considered early in the process, to avoid problems later during attempts to integrate data sets. Note that just beca... (click for more)
Tags: analyze assure database integrate quality tabular
Develop a quality assurance and quality control planJust as data checking and review are important components of data management, so is the step of documenting how these tasks were accomplished. Creating a plan for how to review the data before it is collected or compiled allows a researcher to think sys... (click for more)
Tags: assure data consistency flag measurement quality
Double-check the data you enterEnsuring accuracy of your data is critical to any analysis that follows. (click for more)
Tags: assure data consistency quality
Ensure basic quality controlQuality control practices are specific to the type of data being collected, but some generalities exist: (click for more)
Ensure datasets used are reproducibleWhen searching for data, whether locally on one’s machine or in external repositories, one may use a variety of search terms. In addition, data are often housed in databases or clearinghouses where a query is required in order access data. In order to r... (click for more)
Tags: analyze assure data archives data processing discover provenance replicable data
Identify missing values and define missing value codesMissing values should be handled carefully to avoid their affecting analyses. The content and structure of data tables are best maintained when consistent codes are used to indicate that a value is missing in a data field. Commonly used approaches for c... (click for more)
Tags: assure coding missing values
Identify outliersOutliers may not be the result of actual observations, but rather the result of errors in data collection, data recording, or other parts of the data life cycle. The following can be used to identify outliers for closer examination: (click for more)
Tags: analyze annotation assure quality
Identify values that are estimatedData tables should ideally include values that were acquired in a consistent fashion. However, sometimes instruments fail and gaps appear in the records. For example, a data table representing a series of temperature measurements collected over time fro... (click for more)
Tags: analyze assure flag quality
Mark data with quality control flagsAs part of any review or quality assurance of data, potential problems can be categorized systematically. For example data can be labeled as 0 for unexamined, -1 for potential problems and 1 for “good data.” Some research communities have developed stan... (click for more)
Tags: assure coding data quality flag
Provide version information for use and discoveryProvide versions of data products with defined identifiers to enable discovery and use. (click for more)